ECG signal denoising through kernel principal components
Abstract:
Heart electrical activity is measured on the body surface; this measure is known as electrocardiogram (ECG). The ECG signals are commonly accompanied by different types of noise, that can lead to a difficult and imprecise computational process to diagnose heart diseases. In this paper, we propose the Kernel Principal Component Analysis (KPCA) method, usually used in image denoising, for minimizing the noise presented in ECG signals. The aim is to present a high-performance ECG signal denoising process. We also include a denoising benchmark among Discrete Wavelet Transform, Principal Component Analysis, and KPCA methods. Physionet database was used and the accuracy was realized with the Mean Squared Error (MSE) metric. The lower MSE values were obtained in the majority of cases with the KPCA method, considering signals with SNR of 5dB.
Año de publicación:
2017
Keywords:
- Principal Component Analysis
- kernel principal component analysis
- ECG signal denoising
- Discrete Wavelet Transform
Fuente:
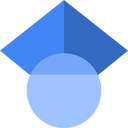
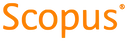
Tipo de documento:
Conference Object
Estado:
Acceso restringido
Áreas de conocimiento:
- Procesamiento de señales
Áreas temáticas:
- Farmacología y terapéutica
- Fisiología humana
- Enfermedades