EEG signal clustering for motor and imaginary motor tasks on hands and feet
Abstract:
Modern technologies use Brain Computer Interfaces (BCI) to control devices or prosthesis for people with physical impairments. In some cases, EEG data are used to determine the intentionality of the subject when performing motor and imaginary motor tasks. However, EEG signals are very susceptible to noise due to the lower voltage levels that are acquired. We used a data set of 64 EEG recordings of 25 healthy subjects while they were doing motor and imaginary motor movements of hands and feet. Data were preprocessing, including the design of a filter for noise reduction outside the expected frequency spectral that operate the EEG signals. Then, we used features extraction based on spectral density. Finally, the application of five clustering algorithms to detect motor and imaginary motor tasks. Results showed that the k-means, k-medoids and Hierarchical clustering algorithms were better detecting motor activity, and hierarchical clustering for imaginary tasks of hands.
Año de publicación:
2017
Keywords:
- k-medoids
- electroencephalography
- DBscan
- Spectral clustering
- Direct Current Artifacts
- kmeans
- power spectral density
- Butterworth Filter
- Fast fourier transform
- hierarchical-clustering
- Brain computer interface
Fuente:
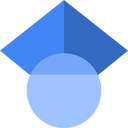
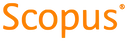
Tipo de documento:
Conference Object
Estado:
Acceso restringido
Áreas de conocimiento:
- Aprendizaje automático
Áreas temáticas:
- Fisiología humana
- Enfermedades