ERISNet: Deep neural network for sargassum detection along the coastline of the mexican caribbean
Abstract:
Recently, Caribbean coasts have experienced atypical massive arrivals of pelagic Sargassum with negative consequences both ecologically and economically. Based on deep learning techniques, this study proposes a novel algorithm for floating and accumulated pelagic Sargassum detection along the coastline of Quintana Roo, Mexico. Using convolutional and recurrent neural networks architectures, a deep neural network (named ERISNet) was designed specifically to detect these macroalgae along the coastline through remote sensing support. A new dataset which includes pixel values with and without Sargassum was built to train and test ERISNet. Aqua-MODIS imagery was used to build the dataset. After the learning process, the designed algorithm achieves a 90% of probability in its classification skills. ERISNet provides a novel insight to detect accurately algal blooms arrivals.
Año de publicación:
2019
Keywords:
- SARGASSUM
- Algal blooms
- Neural networks
- deep learning
- MÉXICO
- remote sensing
- Modis
Fuente:
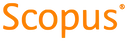
Tipo de documento:
Article
Estado:
Acceso abierto
Áreas de conocimiento:
- Aprendizaje automático
- Ciencias de la computación
- Sensores remotos
Áreas temáticas:
- Ciencias de la computación
- Religión clásica (religión griega y romana)
- Física aplicada