Early Detection of Main Bearing Damage in Wind Turbines
Abstract:
According to the European Wind Energy Academy (EAWE), the wind industry has recognized that main bearing failures are a major concern in order to increase the reliability and availability of wind turbines. This is due to the high replacement cost of major repairs and the long downtime associated with main bearing failures. As a result, pbkp_redicting main bearing failure has become an economically important problem as well as a technological difficulty. This paper presents a data-driven technique based on a closed recurrent unit (GRU) neural network for early failure pbkp_rediction (months in advance). The main contributions of this work are: (i) The pbkp_rediction is made exclusively using SCADA (Supervision Control and Data Acquisition) data already present in all industrial wind turbines. Therefore, there is no need to add additional sensors intended for a specific use. (ii) Since the proposed approach only requires healthy data, it can be used in any wind farm even if it has not recorded faulty data. (iii) The suggested algorithm operates under a variety of operational and environmental circumstances. (iv) The methodology is validated in two real in-production wind turbines. production.
Año de publicación:
2022
Keywords:
- Fault Detection
- SCADA data
- GRU neural networks
- Main bearing
- Wind turbine
Fuente:
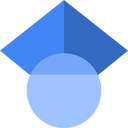
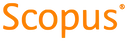
Tipo de documento:
Article
Estado:
Acceso abierto
Áreas de conocimiento:
- Energía
- Ingeniería mecánica
Áreas temáticas de Dewey:
- Física aplicada