Early Fault Diagnosis Strategy for WT Main Bearings Based on SCADA Data and One-Class SVM
Abstract:
To reduce the levelized cost of wind energy, through the reduction in operation and maintenance costs, it is imperative that the wind turbine downtime is reduced through maintenance strategies based on condition monitoring. The standard approach toward this challenge is based on vibration monitoring, which requires the installation of specific tailored sensors that incur associated added costs. On the other hand, the life expectancy of wind parks built during the 1990s wind power boom is dwindling, and data-driven maintenance strategies issued from already accessible supervisory control and data acquisition (SCADA) data is an auspicious competitive solution because no additional sensors are required. Note that it is a major issue to provide fault diagnosis approaches built only on SCADA data, as these data were not established with the objective of being used for condition monitoring but rather for control capacities. The present study posits an early fault diagnosis strategy based exclusively on SCADA data and supports it with results on a real wind park with 18 wind turbines. The contributed methodology is an anomaly detection model based on a one-class support vector machine classifier; that is, it is a semi-supervised approach that trains a decision function that categorizes fresh data as similar or dissimilar to the training set. Therefore, only healthy (normal operation) data is required to train the model, which greatly expands the possibility of employing this methodology (because there is no need for faulty data from the past, and only normal operation SCADA data is needed). The results obtained from the real wind park show that this is a promising strategy.
Año de publicación:
2022
Keywords:
- condition monitoring
- Condition-Based Maintenance
- Wind turbine
- Anomaly detection
- Main bearing
- SCADA data
- pbkp_redictive maintenance
- One-Class Support Vector Machine
- Fault diagnosis
Fuente:
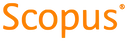
Tipo de documento:
Article
Estado:
Acceso abierto
Áreas de conocimiento:
- Aprendizaje automático
Áreas temáticas:
- Métodos informáticos especiales
- Física aplicada