Effectiveness of causality-based pbkp_redictor selection for statistical downscaling: a case study of rainfall in an Ecuadorian Andes basin
Abstract:
Downscaling aims to take large-scale information and map it to smaller scales to reproduce local climate signals. An essential step in implementing a parsimonious downscaling model is the selection of a subset of relevant pbkp_redictors that increase the simulation accuracy. According to relevant literature, pbkp_redictive models that use pbkp_redictors chosen through causality methods, not widely used in downscaling, are more interpretable and robust. Characteristics that do not necessarily pursue the selection methods are commonly used in machine learning (ML), where the primary objective is to maximize accuracy. This study then explores whether the improvement in interpretability and robustness of models that use causally selected pbkp_redictors is not achieved at the expense of poor downscaling performance in terms of simulation accuracy. To this end, one set of three methods based on causality and one set of four standard pbkp_redictor selection methods used in ML are compared, centered on the downscaling performance of rainfall in an Ecuadorian Andes basin. One causality-based selection method is based on Granger causality (GC), and two are based on a constraint-based algorithm for structure learning, namely the Peter and Clark algorithm (PCalg) and the based on PCalg and the momentary conditional independence (PCMCI). Regarding the methods used in ML, one is based on the variance of the pbkp_redictors (variance threshold), two are based on univariate statistical tests (F test and mutual information), and one is based on the recursive elimination of pbkp_redictors (RFE) according to their contribution to the pbkp_rediction model. The number of methods in each set results from those that are considered standard in many ML libraries or have been used for regression or climatology (causality-based). The selected pbkp_redictors are used to train statistical downscaling models based on the random forest and support vector machine learning algorithms to assess their performance and rank the selection methods. Among the most remarkable findings, the methods based on causality show a robust selection. For instance, the causality-based methods selected 53 different pbkp_redictors in one of the considered stations, while the ML-based methods selected 73 for downscaling models with similar performance. Furthermore, when the pbkp_redictors used in the best performing downscaling models are analyzed, it is shown that the different selection methods based on causality tend to choose a subset of pbkp_redictors that do not differ greatly, contrary to the methods based on machine learning. This evidences a more concise selection of the causality-based methods. Finally, the evaluation metrics of the downscaling models showed that the best selection method is RFE, but the following best were consistently those based on causality (mainly PCMCI). The study evidences no significant performance detriment when using the causally selected pbkp_redictors.
Año de publicación:
2022
Keywords:
Fuente:
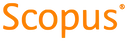
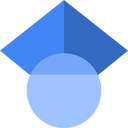
Tipo de documento:
Article
Estado:
Acceso restringido
Áreas de conocimiento:
- Inferencia estadística
- Inferencia estadística
Áreas temáticas:
- Geología, hidrología, meteorología
- Topología
- Agricultura y tecnologías afines