Effects of Data Augmentation on the Identification of Cough Sound Using Convolutional Neural Networks
Abstract:
Cough identification employing deep learning models has generated significant interest in fields such as telemedicine. However, it is challenging to have a considerable number of cough sounds to train such models, and consequently, it is necessary to apply techniques to generate more data. In this work, the effect of data augmentation on the identification of cough was studied using convolutional neural networks (CNN). For this, a system composed of Front-End and Back-End was implemented. In the Front-End, the original recordings and those generated by data augmentation by frequency shift and noise injection were processed and transformed into Mel spectrograms. On the Back-End, the spectrograms were analyzed and classified using a CNN. The results show that the transformations proposed to increase the amount of data help reducing the overfitting by 42.5% while bettering accuracy by a relative improvement of 1%. Therefore, applying data augmentation techniques to train CNNs for differentiating coughs from other sound events results in a simple but effective strategy.
Año de publicación:
2022
Keywords:
- convolutional neural networks
- Cough identification
- data augmentation
Fuente:
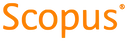
Tipo de documento:
Conference Object
Estado:
Acceso restringido
Áreas de conocimiento:
- Aprendizaje automático
- Ciencias de la computación
Áreas temáticas:
- Ciencias de la computación