Efficient model partitioning for distributed model transformations
Abstract:
As the models that need to be handled in model-driven engineering grow in scale, scalable algorithms for model transformation (MT) are becoming necessary. Programming models such as MapReduce or Pregel may simplify the development of distributed model transformations. However, because of the dense inter-connectivity of models and the complexity of transformation logics, scalability in distributed model processing is challenging. In this paper, we adapt existing formalization of uniform graph partitioning to the case of distributed MTs by means of binary linear programming. Moreover, we propose a data distribution algorithm for declarative model transformation based on static analysis of relational transformation rules. We first extract footprints from transformation rules. Then we propose a fast data distribution algorithm, driven by the extracted footprints, and based on recent results on balanced partitioning of streaming graphs. To validate our approach, we apply it to an existing distributed MT engine for the ATL language, built on top of MapReduce. We implement our heuristic as a custom split algorithm for ATL on MapReduce and we evaluate its impact on remote access to the underlying backend.
Año de publicación:
2016
Keywords:
- Static Analysis
- mapreduce
- Data distribution
- ATL
- Model transformation
Fuente:
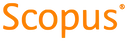
Tipo de documento:
Conference Object
Estado:
Acceso restringido
Áreas de conocimiento:
- Ingeniería de software
- Optimización matemática
Áreas temáticas:
- Programación informática, programas, datos, seguridad
- Métodos informáticos especiales
- Ciencias de la computación