Efficient processing of semantically represented sensor data
Abstract:
Large collections of sensor data are semantically described using ontologies, e.g., the Semantic Sensor Network (SSN) ontology. Semantic sensor data are RDF descriptions of sensor observations from related sampling frames or sensors at multiple points in time, e.g., climate sensor data. Sensor values can be repeated in a sampling frame, e.g., a particular temperature value can be repeated several times, resulting in a considerable increase in data volume. We devise a factorized compact representation of semantic sensor data using linked data technologies to reduce repetition of same sensor values, and propose algorithms to generate collections of factorized semantic sensor data that can be managed by existing RDF triple stores. We empirically study the effectiveness of the proposed factorized representation of semantic sensor data. We show that the size of semantic sensor data is reduced by more than 50% on average without loss of information. Further, we have evaluated the impact of this factorized representation of semantic sensor data on query execution. Results suggest that query optimizers can be empowered with semantics from factorized representations to generate query plans that effectively speed up query execution time on factorized semantic sensor data.
Año de publicación:
2017
Keywords:
- Data factorization
- Sensor
- Linked data
- Query optimization and execution
Fuente:
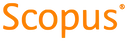
Tipo de documento:
Conference Object
Estado:
Acceso restringido
Áreas de conocimiento:
- Sensor
- Ciencias de la computación
- Software
Áreas temáticas:
- Ciencias de la computación