Alignment-Free Antimicrobial Peptide Pbkp_redictors: Improving Performance by a Thorough Analysis of the Largest Available Data Set
Abstract:
In the last two decades, a large number of machine-learning-based pbkp_redictors for the activities of antimicrobial peptides (AMPs) have been proposed. These pbkp_redictors differ from one another in the learning method and in the training and testing data sets used. Unfortunately, the training data sets present several drawbacks, such as a low representativeness regarding the experimentally validated AMP space, and duplicated peptide sequences between negative and positive data sets. These limitations give a low confidence to most of the approaches to be used in prospective studies. To address these weaknesses, we propose novel modeling and assessing data sets from the largest experimentally validated nonredundant peptide data set reported to date. From these novel data sets, alignment-free quantitative sequence-activity models (AF-QSAMs) based on Random Forest are created to identify general AMPs and their antibacterial, antifungal, antiparasitic, and antiviral functional types. An applicability domain analysis is carried out to determine the reliability of the pbkp_redictions obtained, which, to the best of our knowledge, is performed for the first time for AMP recognition. A benchmarking is undertaken between the models proposed and several models from the literature that are freely available in 13 programs (ClassAMP, iAMP-2L, ADAM, MLAMP, AMPScanner v2.0, AntiFP, AMPfun, PEPred-suite, AxPEP, CAMPR3, iAMPpred, APIN, and Meta-iAVP). The models proposed are those with the best performance in all of the endpoints modeled, while most of the methods from the literature have weak-to-random pbkp_redictive agreements. The models proposed are also assessed through Y-scrambling and repeated k-fold cross-validation tests, demonstrating that the outcomes obtained by them are not given by chance. Three chemometric analyses also confirmed the relevance of the peptides descriptors used in the modeling. Therefore, it can be concluded that the models built by fixing the drawbacks existing in the literature contribute to identifying antibacterial, antifungal, antiparasitic, and antiviral peptides with high effectivity and reliability. Models are freely available via the AMPDiscover tool at https://biocom-ampdiscover.cicese.mx/.
Año de publicación:
2021
Keywords:
Fuente:
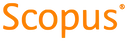
Tipo de documento:
Article
Estado:
Acceso restringido
Áreas de conocimiento:
- Resistencia antimicrobiana
- Microbiología
Áreas temáticas:
- Ciencias de la computación
- Biología
- Ciencias sociales