Alignment-free methods for the detection and specificity pbkp_rediction of adenylation domains
Abstract:
Identifying adenylation domains (A-domains) and their substrate specificity can aid the detection of nonribosomal peptide synthetases (NRPS) at genome/proteome level and allow inferring the structure of oligopeptides with relevant biological activities. However, that is challenging task due to the high sequence diversity of A-domains (~10–40% of amino acid identity) and their selectivity for 50 different natural/unnatural amino acids. Altogether these characteristics make their detection and the pbkp_rediction of their substrate specificity a real challenge when using traditional sequence alignment methods, e.g., BLAST searches. In this chapter we describe two workflows based on alignment-free methods intended for the identification and substrate specificity pbkp_rediction of A-domains. To identify A-domains we introduce a graphical-numerical method, implemented in TI2BioP version 2.0 (topological indices to biopolymers), which in a first step uses protein four-color maps to represent A-domains. In a second step, simple topological indices (TIs), called spectral moments, are derived from the graphical representations of known A-domains (positive dataset) and of unrelated but well-characterized sequences (negative set). Spectral moments are then used as input pbkp_redictors for statistical classification techniques to build alignment-free models. Finally, the resulting alignment-free models can be used to explore entire proteomes for unannotated A-domains. In addition, this graphical-numerical methodology works as a sequence-search method that can be ensemble with homology-based tools to deeply explore the A-domain signature and cope with the diversity of this class (Aguero-Chapin et al., PLoS One 8(7):e65926, 2013). The second workflow for the pbkp_rediction of A-domain’s substrate specificity is based on alignment-free models constructed by transductive support vector machines (TSVMs) that incorporate information of uncharacterized A-domains. The construction of the models was implemented in the NRPSpbkp_redictor and in a first step uses the physicochemical fingerprint of the 34 residues lining the active site of the phenylalanine-adenylation domain of gramicidin synthetase A [PDB ID 1 amu] to derive a feature vector. Homologous positions were extracted for A-domains with known and unknown substrate specificities and turned into feature vectors. At the same time, A-domains with known specificities towards similar substrates were clustered by physicochemical properties of amino acids (AA). In a second step, support vector machines (SVMs) were optimized from feature vectors of characterized A-domains in each of the resulting clusters. Later, SVMs were used in the variant of TSVMs that integrate a fraction of uncharacterized A-domains during training to pbkp_redict unknown specificities. Finally, uncharacterized A-domains were scored by each of the constructed alignment-free models (TSVM) representing each substrate specificity resulting from the clustering. The model producing the largest score for the uncharacterized A-domain assigns the substrate specificity to it (Rausch et al., Nucleic Acids Res 33:5799–5808, 2005).
Año de publicación:
2016
Keywords:
- Transductive support vector machines
- topological indices
- NRPS
- Adenylation domains
- Alignment-free models
Fuente:
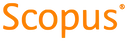
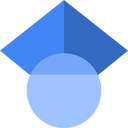
Tipo de documento:
Book Part
Estado:
Acceso restringido
Áreas de conocimiento:
- Biología molecular
Áreas temáticas:
- Programación informática, programas, datos, seguridad
- Biología
- Fisiología humana