All-Analog Silicon Integration of Image Sensor and Neural Computing Engine for Image Classification
Abstract:
We have designed a fully-integrated analog CMOS cognitive image sensor based on a two-layer artificial neural network and targeted to low-resolution image classification. We have used a single poly 180 nm CMOS process technology, which includes process modules for realizing the building blocks of the CMOS image sensor. Our design includes all the analog sub-circuits required to perform the cognitive sensing task, from image sensing to output classification decision. The weights of the network are stored in single-poly floating-gate memory cells, using a single transistor per analog weight. This enables the classifier to be intrinsically reconfigurable, and to be trained for various classification problems, based on low-resolution images. As a case study, the classifier capability is tested using a low-resolution version of the MNIST dataset of handwritten digits. The circuit exhibits a classification accuracy of 87.8%, that is comparable to an equivalent software implementation operating in the digital domain with floating point data precision, with an average energy consumption of 6 nJ per inference, a latency of 22.5 mu text{s} and a throughput of up to 133.3 thousand inferences per second.
Año de publicación:
2022
Keywords:
- Analog neural network
- neuromorphic engineering
- cognitive image sensor
Fuente:
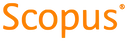
Tipo de documento:
Article
Estado:
Acceso abierto
Áreas de conocimiento:
- Red neuronal artificial
- Ingeniería electrónica
Áreas temáticas:
- Física aplicada
- Ingeniería y operaciones afines
- Métodos informáticos especiales