Alternative Ensemble Classifier Based on Penalty Strategy for Improving Pbkp_rediction Accuracy
Abstract:
The Increasing demand for accurate classifier systems for user’s service has called the application of machine learning techniques. One of the most used techniques consist in grouping classifiers into an ensemble classifier. The resulting classifier is generally more accurate than any individual classifier. In this work, we propose an alternative ensemble classification system based on combining three classifiers: Naive Bayes, Random Forest and Multilayer Perceptron. To increase robustness of pbkp_rediction, we organized the algorithms used by penalty calculations instead of a score-based voting system. We have compared the results of our proposed penalty factor system with the most popular classification algorithms and an ensemble classifier that uses the voting technique. Our results show that our algorithm improves the accuracy in pbkp_rediction of classification in exchange of a reasonable response time.
Año de publicación:
2019
Keywords:
- Machine learning
- classification algorithm
- Ensemble classification
- classification
Fuente:
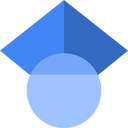
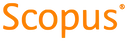
Tipo de documento:
Conference Object
Estado:
Acceso restringido
Áreas de conocimiento:
- Aprendizaje automático
- Algoritmo
Áreas temáticas de Dewey:
- Programación informática, programas, datos, seguridad