Alzheimer’s disease diagnosis system using electroencephalograms and machine learning models
Abstract:
Alzheimer’s disease (AD) is a neurodegenerative disorder that gradually destroys brain cells causing loss of memory and cognitive abilities. The potential of Electroencephalograms (EEG) for the diagnosis of AD is currently studied since it is a low-cost, non-invasive technique that might be very useful for an early diagnosis. This research aims to propose a system for AD detection using characteristics extracted from the EEG and the best classification model. To this end, a comparative study is carried out using characteristics that capture two effects that AD produces on EEGs, which are the slowing and the complexity reduction of the signal. Different classification models are compared, such as Feed-Forward Neural Networks (FFNN), Support Vector Machines, among others. The best features were Higuchi’s fractal dimension and Power Spectral Density and the best classifier was FFNN. The proposed method, classifying between AD patients and healthy controls, achieves an accuracy of 99.8%.
Año de publicación:
2020
Keywords:
- feature selection
- Feed forward neural networks
- Alzheimer’s disease
- Electroencephalograms
Fuente:
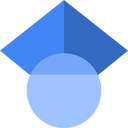
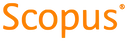
Tipo de documento:
Article
Estado:
Acceso restringido
Áreas de conocimiento:
- Neurología
- Aprendizaje automático
- Ciencias de la computación
Áreas temáticas de Dewey:
- Medicina y salud
- Enfermedades
- Física aplicada