Elderly fall detection using data classification on a portable embedded system
Abstract:
The area of research on the detection of falls in the elderly allows to prevent major ailments to a person and not receiving timely medical attention. Although different systems have been proposed for the detection of falls, there are some open problems such as: cost, computational load, precision, portability, among others. This paper presents an alternative approach based on the acquisition of speed variation of the person on the X, Y and Z axes using an accelerometer and machine learning techniques. Since the information acquired by the sensor is very variant, with noise and high volume of data, a prototype selection stage is carried out using confidence intervals and techniques of Leaving-One-Out. Subsequently, automatic detection is performed using the K-nearest neighbors (K-NN) classifier. As a result of fall detection 95% accuracy is achieved in experiments from 5 trials and already used in reality by an older adult, the system has a time of 30 ms for position selection and the detection of drop is maintained in a 92% right.
Año de publicación:
2017
Keywords:
- Fall detection
- Embedded System
- Knn
- prototype selection
Fuente:
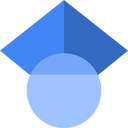
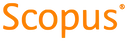
Tipo de documento:
Conference Object
Estado:
Acceso restringido
Áreas de conocimiento:
- Ciencias de la computación
- Sistema embebido
Áreas temáticas:
- Ciencias de la computación
- Medicina y salud
- Física aplicada