Emerging Computational Approaches for Antimicrobial Peptide Discovery
Abstract:
In the last two decades many reports have addressed the application of artificial intelligence (AI) in the search and design of antimicrobial peptides (AMPs). AI has been represented by machine learning (ML) algorithms that use sequence-based features for the discovery of new peptidic scaffolds with promising biological activity. From AI perspective, evolutionary algorithms have been also applied to the rational generation of peptide libraries aimed at the optimization/design of AMPs. However, the literature has scarcely dedicated to other emerging non-conventional in silico approaches for the search/design of such bioactive peptides. Thus, the first motivation here is to bring up some non-standard peptide features that have been used to build classical ML pbkp_redictive models. Secondly, it is valuable to highlight emerging ML algorithms and alternative computational tools to pbkp_redict/design AMPs as well as to explore their chemical space. Another point worthy of mention is the recent application of evolutionary algorithms that actually simulate sequence evolution to both the generation of diversity-oriented peptide libraries and the optimization of hit peptides. Last but not least, included here some new considerations in proteogenomic analyses currently incorporated into the computational workflow for unravelling AMPs in natural sources.
Año de publicación:
2022
Keywords:
- Artificial Intelligence
- Machine learning
- complex networks
- Evolutionary algorithms
- Amps
- Molecular descriptors
- proteogenomics
Fuente:
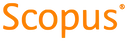
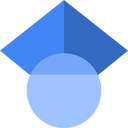
Tipo de documento:
Review
Estado:
Acceso abierto
Áreas de conocimiento:
- Biotecnología
- Farmacología
Áreas temáticas:
- Programación informática, programas, datos, seguridad
- Medicina forense; incidencia de enfermedades
- Fisiología y materias afines