End-To-end Deep Learning for VCSEL's Nonlinear Digital Pre-Distortion
Abstract:
We propose a novel optimization method for a Neural Network based Digital Pre-Distorter (DPD), applied in Intensity Modulation-Direct Detection transmission systems leveraging Multi-Modal Fiber and Vertical-Cavity Surface-Emitting Laser. We train the DPD using End-To-end Deep Learning of the optical link, together with a Direct Learning Approach leveraging experimental measurements for modeling the transmission channel. The optimization considers VCSEL amplitude constraints, the use of an FFE at the receiver side, and the presence of a receiver non-flat Colored Gaussian Noise (CGN). We verify our optimized DPD on an experimental setup transmitting a 92 Gbps PAM-4 modulated signal. We achieve, for BER=0.01, a performance gain of more than 1 dB in terms of Optical Path Loss with respect to the best performing non-pre-distorted scenario.
Año de publicación:
2022
Keywords:
- nonlinear equalization
- Deep learning applications on communication systems
- VCSEL
- Optical PAM-4 IM-DD systems
- Data center interconnects
Fuente:
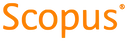
Tipo de documento:
Conference Object
Estado:
Acceso restringido
Áreas de conocimiento:
- Algoritmo
- Aprendizaje profundo
- Óptica no lineal
Áreas temáticas:
- Métodos informáticos especiales