Energy Consumption of a Building by using Long Short-Term Memory Network: A Forecasting Study
Abstract:
Buildings have a dominant presence in energy consumption for the transition to clean energy. During 2017, construction and operation of buildings worldwide represented more than a third (36%) of final energy used and 40% of the emissions of carbon dioxide. Hence, there is great interest in reducing energy use in this sector, and energy efficiency in buildings to enhance energy performances is a suitable way. In this paper, black-box approaches based on artificial neural networks to pbkp_redict the electric load of a selected educational building are proposed the potential and robustness of long short-Term memory (LSTM) applied to a dataset with a limited number of days of observations are analyzed the results in our scenario showed that the LSTM surpasses in accuracy to other techniques such as feed-forward neural networks.
Año de publicación:
2020
Keywords:
- electric load time series
- energy efficiency building
- Feed-forward neural networks
- long short-Term memory
Fuente:
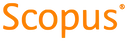
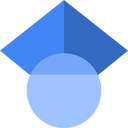
Tipo de documento:
Conference Object
Estado:
Acceso restringido
Áreas de conocimiento:
- Aprendizaje automático
- Energía
- Simulación por computadora
Áreas temáticas de Dewey:
- Ciencias de la computación
- Física aplicada
- Métodos informáticos especiales