Enhanced replacement method integration with genetic algorithms populations in QSAR and QSPR theories
Abstract:
The selection of an optimal set of molecular descriptors from a much larger collection of such regression variables is a vital step in the elaboration of most QSAR and QSPR models. The aim of this work is to continue advancing this important selection process by combining the enhanced replacement method (ERM) and the well-known genetic algorithms (GA). These approaches had previously proven to yield near-optimal results with a much smaller number of linear regressions than a full search. The newly proposed algorithms were tested on four different experimental datasets, formed by collections of 116, 200, 78, and 100 experimental records from different compounds and 1268, 1338, 1187, and 1306 molecular descriptors, respectively. The comparisons showed that the new alternative ERMp (combination of ERM with a GA population) further improves ERM, it has previously been shown that the latter is superior to GA for the selection of an optimal set of molecular descriptors from a much greater pool.
Año de publicación:
2015
Keywords:
- Enhanced replacement method
- QSPR
- QSAR
- Genetic Algorithm
Fuente:
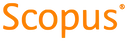
Tipo de documento:
Article
Estado:
Acceso restringido
Áreas de conocimiento:
- Relación cuantitativa estructura-actividad
- Genética
- Aprendizaje automático
Áreas temáticas:
- Química física