Ensemble Kalman Filter and Extended Kalman Filter for State-Parameter Dual Estimation in Mixed Effects Models Defined by a Stochastic Differential Equation
Abstract:
The biological processes that occur in the real world have complex dynamics. Mathematical models that try to describe these phenomena have nonlinear structures, observations are made at discrete time points and include measurement errors, and are difficult to estimate. In particular, when modeling dynamics of repeated measurements on individuals or objects, they are analyzed by mixed-effects diffusion models. The standard estimation methods in these cases are: maximum likelihood, EM, SAEM, Newton Raphson, among others. In this paper we propose a specific inference methodology for models. Apply extended Kalman Filter (EKF) and the ensemble Kalman filter (EnKF) to the estimation of both states and parameters of nonlinear state-space models. To illustrate the methodology, the states and parameters of an Ornstein- Uhlembeck (O-U) mixed-effects model were estimated, obtaining precise estimates with small standard deviations. To measure the estimation quality of the algorithms was used as a measure of goodness-of-fit known as the square root of the mean quadratic error, obtaining very small errors.
Año de publicación:
2020
Keywords:
- Mixed effects models
- Dual extended Kalman filter
- Dual ensemble Kalman filter
- Stochastic differential equation
Fuente:
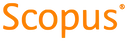
Tipo de documento:
Conference Object
Estado:
Acceso restringido
Áreas de conocimiento:
- Optimización matemática
- Proceso estocástico
- Aprendizaje automático
Áreas temáticas de Dewey:
- Ciencias de la computación

Objetivos de Desarrollo Sostenible:
- ODS 3: Salud y bienestar
- ODS 17: Alianzas para lograr los objetivos
- ODS 9: Industria, innovación e infraestructura
