Ensemble Learning Models Applied in Energy Time Series of a University Building
Abstract:
During 2020, the construction and operation of buildings globally accounted for more than a third (36%) of final energy used and 37% of carbon dioxide emissions. Hence, in the last decade, there has been a great interest in analyzing energy efficiency in buildings from different approaches. In this paper, machine learning-based black-box methods are proposed to pbkp_redict the energy consumption of a university building. In related works, little has been explored on machine learning techniques such as decision trees and ensemble learning for pbkp_redicting time series of energy consumption in homes and buildings. Hence, this work proposes an analysis of forecast accuracy and computational times of decision tree and ensemble learning techniques such as Random Forest and Extreme Gradient Boosting applied particularly to time series of the total active power of a university building. The results show that Random Forest presents the best forecast error metrics RMSE, MAE, and MAPE. However, this model ranks second in computational time, below the decision tree technique. These models can be beneficial in pbkp_redictive control systems considered in buildings to forecast the behavior of buildings' energy consumption in the short term with outstanding precision.
Año de publicación:
2022
Keywords:
- Energy consumption
- random forest
- Decision Trees
- ensemble learning
- Cross-validation for time series
- Extreme Gradient Boosting
Fuente:
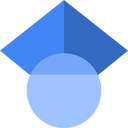
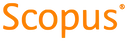
Tipo de documento:
Conference Object
Estado:
Acceso restringido
Áreas de conocimiento:
- Energía
- Energía
- Estadísticas
Áreas temáticas de Dewey:
- Física aplicada
- Ingeniería y operaciones afines
- Otras ramas de la ingeniería