Ensemble Learning aided QPSO–Based Framework for Secrecy Energy Efficiency in FD CR-NOMA Systems
Abstract:
Cognitive radio (CR), non-orthogonal multiple access (NOMA), and full-duplex (FD) communications have been considered key technologies for providing spectrum utilization improvement and higher energy efficiency on the internet of things (IoT) networks and next-generation communication. However, security concerns are still an issue to be addressed because confidential information is exposed in wireless systems. To solve this problem, we design a novel artificial intelligence (AI)-based framework for maximizing the secrecy energy efficiency (SEE) in FD cooperative relay underlay CR-NOMA systems that are exposed to multiple eavesdroppers. First, we formulate the non-convex SEE optimization problem as bi-level optimization, subject to constraints that satisfy the quality-of-service requirements of secondary users. In particular, the outer problem is solved with ensemble learning (EL) to select the optimal relay. Regarding the inner problem, we propose a quantum particle swarm optimization (QPSO)-based technique to optimize power allocation. In addition, for comparison purposes, we describe a cooperative relay CR network with orthogonal multiple access (OMA), rate-splitting multiple access (RSMA), and half-duplex technologies. Moreover, we evaluate comparative schemes based on machine learning algorithms and swarm intelligence baseline schemes. Furthermore, the proposed EL-aided QPSO-based framework achieves performance close to the optimal solutions, with a meaningful reduction in computation complexity.
Año de publicación:
2022
Keywords:
- Particle Swarm Optimization
- quantum particular swarm optimization (QPSO)
- Cognitive Radio (CR)
- ensemble learning
- security
- Optimization
- Resource Management
- Relays
- NOMA
- wireless networks
- Secrecy energy efficiency (SEE)
- Non-Orthogonal Multiple Access (NOMA)
Fuente:
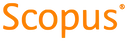
Tipo de documento:
Article
Estado:
Acceso restringido
Áreas de conocimiento:
- Aprendizaje automático
- Simulación por computadora
Áreas temáticas:
- Ciencias de la computación