An Adaptive Strategy for Medium-Term Electricity Consumption Forecasting for Highly Unpbkp_redictable Scenarios: Case Study Quito, Ecuador during the Two First Years of COVID-19
Abstract:
This research focuses its efforts on the pbkp_rediction of medium-term electricity consumption for scenarios of highly variable electricity demand. Numerous approaches are used to pbkp_redict electricity demand, among which the use of time series (ARMA, ARIMA) and the use of machine learning techniques, such as artificial neural networks, are the most covered in the literature review. All these approaches evaluate the pbkp_rediction error when comparing the generated models with the data that fed the model, but they do not compare these values with the actual data of electricity demand once these are obtained, in addition, these techniques present high error values when there are unexpected changes in the trend of electricity consumption. This work proposes a methodology to generate an adaptive model for unexpected changes in electricity demand through the use of optimization in conjunction with (Formula presented.) time series. The proposed case study is the electricity consumption in Quito, Ecuador to pbkp_redict the electricity demand in the years 2019 and 2020, which are particularly challenging due to atypical electricity consumption attributed to COVID-19. The results show that the proposed model is capable of following the trend of electricity demand, adapting itself to sudden changes and obtaining an average error of 2.5% which is lower than the average error of 5.43% when using a non-adaptive approach (more than 50% or error improvement).
Año de publicación:
2022
Keywords:
- Power demand
- load forecasting
- Demand Forecasting
- Optimization techniques
- adaptive models
- medium term forecasting
- time series analysis
Fuente:
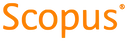
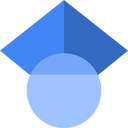
Tipo de documento:
Article
Estado:
Acceso abierto
Áreas de conocimiento:
- Energía
- Energía
Áreas temáticas:
- Física aplicada
- Ciencias de la computación
- Economía de la tierra y la energía