An Analysis of Deep Learning Architectures for Cancer Diagnosis
Abstract:
It was analyzed the reference information on Deep Learning applications in the areas of diagnosis and pbkp_rediction of different types of cancer. The problem is to perform the analysis and obtain the criteria to select a Deep Learning architecture for cancer diagnosis. The objective is to carry out an analysis of Deep Learning architectures and select a model to apply training and tests that assist in the diagnosis of cancer. It was used as a method the exploratory research and deduction to analyze the reference information on Deep Learning theories and architectures applied in cancer diagnosis; it also describes the reasons for selecting a model, scope, proposal, configuration parameters and structure for a CNN network. It resulted in the Impact of Deep Learning in cancer diagnosis, Training the CNN network, and Testing the CNN network. It was concluded that the 9-layer CNN Simple model used for training and testing on a data set of 8801 breast cancer images, has good properties and generates quantitative results for image classification; in the adopted model, a precision rate was obtained on the data set that reached 85.67% in training and 85.87% in tests; the quality of the model in classification tasks is 86%; this indicates the good stability and efficiency of the model.
Año de publicación:
2021
Keywords:
- Cancer diagnosis
- deep learning
- architectures
- Machine learning
Fuente:
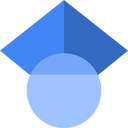
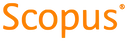
Tipo de documento:
Conference Object
Estado:
Acceso restringido
Áreas de conocimiento:
- Aprendizaje automático
- Cáncer
- Ciencias de la computación
Áreas temáticas:
- Enfermedades
- Medicina y salud
- Ciencias de la computación