An Integrated ARMA-Based Deep Autoencoder and GRU Classifier System for Enhanced Recognition of Daily Hand Activities
Abstract:
Recognition of hand activities of daily living (hand-ADL) is useful in the areas of human-computer interactions, lifelogging, and healthcare applications. However, developing a reliable human activity recognition (HAR) system for hand-ADL with only a single wearable sensor is still a challenge due to hand movements that are typically transient and sporadic. Approaches based on deep learning methodologies to reduce noise and extract relevant features directly from raw data are becoming more promising for implementing such HAR systems. In this work, we present an ARMA-based deep autoencoder and a deep recurrent network (RNN) using Gated Recurrent Unit (GRU) for recognition of hand-ADL using signals from a single IMU wearable sensor. The integrated ARMA-based autoencoder denoises raw time-series signals of hand activities, such that better representation of human hand activities can be made. Then, our deep RNN-GRU recognizes seven hand-ADL based upon the output of the autoencoder: namely, Open Door, Close Door, Open Refrigerator, Close Refrigerator, Open Drawer, Close Drawer, and Drink from Cup. The proposed methodology using RNN-GRU with autoencoder achieves a mean accuracy of 84.94% and F1-score of 83.05% outperforming conventional classifiers such as RNN-LSTM, BRNN-LSTM, CNN, and Hybrid-RNNs by 4-10% higher in both accuracy and F1-score. The experimental results also showed the use of the autoencoder improves both the accuracy and F1-score of each conventional classifier by 12.8% in RNN-LSTM, 4.37% in BRNN-LSTM, 15.45% CNN, 14.6% Hybrid RNN, and 12.4% for the proposed RNN-GRU.
Año de publicación:
2021
Keywords:
- cnn
- Autoregressive processes
- wearable sensors
- rnn
- deep learning
- hand activity recognition
Fuente:
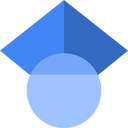
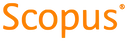
Tipo de documento:
Article
Estado:
Acceso restringido
Áreas de conocimiento:
- Aprendizaje automático
- Ciencias de la computación
Áreas temáticas:
- Ciencias de la computación