An Optimized Soil Moisture Pbkp_rediction Model for Smart Agriculture Using Gaussian Process Regression
Abstract:
An accurate soil moisture model is critical in the design and implementation of a smart agriculture system. Accurate soil moisture pbkp_rediction allows an efficient water resources allocation. This paper presented a soil moisture model using different environmental parameters such as humidity, temperature, light intensity, and rain occurrence as inputs or pbkp_redictor variables. Gaussian process regression algorithm, a non-parametric machine learning algorithm, was used to develop the model. The most effective kernel function was also determined by developing four different GPR models using a different kernel function. In terms of RMSE, the rational quadratic function obtained the lowest value. To further improve the accuracy of the GPR model, an automated hyperparameter tuning was done using a Bayesian optimization algorithm. Three hyperparameters were tuned using the Bayesian optimization algorithm, which improved the GPR model's performance. The optimized GPR model achieved the lowest RMSE and MAE of 3.596 and 1.176, respectively.
Año de publicación:
2022
Keywords:
- Smart agriculture
- Bayesian optimization
- soil moisture
- kernel function
- Machine learning
Fuente:
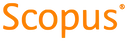
Tipo de documento:
Conference Object
Estado:
Acceso restringido
Áreas de conocimiento:
- Aprendizaje automático
- Optimización matemática
Áreas temáticas:
- Ciencias de la computación
- Agricultura y tecnologías afines
- Técnicas, equipos y materiales