Estimating expected student academic performance
Abstract:
In recent decades, society has a primary need for improving education systems. Pbkp_redicting the performance of students has become a reference topic very analyzed by the research community. Currently, there are several cutting-edge technologies that make very easy to collect educational data in institutional systems due to the new information management systems where almost everything is digitalized. The analysis of this information offers unique opportunities that have a direct impact on students, instructors and academic institutions programs. Concretely, we propose a modular system to evaluate teaching performance by considering several primary factors related to students learning process. In this work, we present the first module, a statistical model that aims at obtaining the expected student’s achievements in a particular course. We first analyzed students’ performance primary factors on Higher Education Systems. To identify these factors, we have conducted a literature review. Then, we examine three different techniques to build the pbkp_rediction model: Multiple Linear Regression (MLR), Support Vector Machine (SVM) and Artificial Neural Networks (ANN). To select the suitable technique, we carried out a variety of experiments by using a real dataset from University of Santa Elena (Ecuador). The achieved experiments revealed promising results.
Año de publicación:
2021
Keywords:
- ACADEMIC PERFORMANCE
- Pbkp_rediction algorithms
- Machine learning
Fuente:
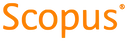
Tipo de documento:
Conference Object
Estado:
Acceso restringido
Áreas de conocimiento:
- Psicología educativa
Áreas temáticas:
- Educación