Estimating relevance of variables for effective recombination
Abstract:
Dominance, extensions of dominance, decomposition, and indicator functions are well-known approaches used to design MOEAs. Algorithms based on these approaches have mostly sought to enhance parent selection and survival selection. In addition, several variation operators have been developed for MOEAs. We focus on the classification and selection of variables to improve the effectiveness of solution search. In this work, we propose a method to classify variables that influence convergence and increase their recombination rate, aiming to improve convergence of the approximation found by the algorithm. We incorporate the proposed method into NSGA-II and study its effectiveness using three-objective DTLZ and WFG functions, including unimodal, multimodal, separable, non-separable, unbiased, and biased functions. We also test the effectiveness of the proposed method on a real-world bi-objective problem. Simulation results verify that the proposed method can contribute to achieving faster and better convergence in several kinds of problems, including the real-world problem.
Año de publicación:
2019
Keywords:
- Evolutionary multi-objective optimization
- Recombination operators
- Variables selection
- Variables classification
Fuente:
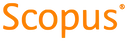
Tipo de documento:
Conference Object
Estado:
Acceso restringido
Áreas de conocimiento:
- Aprendizaje automático
- Optimización matemática
Áreas temáticas:
- Métodos informáticos especiales