Estimation of Ordinary Differential Equations Solutions with Gaussian Processes and Polynomial Chaos Expansion
Abstract:
Derivative modeling is a wide-used technique in the estimation of solutions of systems of differential equations whose numerical solution has an intractable computational complexity or in which the presence of error or infinitesimal perturbations could result in their divergence. The quantification of the uncertainty that is produced when estimating the solution in a finite mesh is an open problem and has been addressed from various probabilistic approaches. In this work, uncertainty estimation of solutions of ordinary differential equations by means of a GP process in a space of smoothed functions is addressed by implementing an algorithm that allows estimating the solution states x(t) and their derivatives in a sequential way. Besides, the addition of polynomial chaos expansions (PCE) using the resulting distributions of the algorithm is proposed to improve the pbkp_rediction of the algorithm. To illustrate the methodology, the algorithms were tested on three known systems of ordinary differential equations and their effectiveness was quantified by three performance measures, resulting in an overall improvement in pbkp_rediction by adding the polynomial chaos expansion.
Año de publicación:
2021
Keywords:
- Polynomial chaos expansion
- Ordinary differential equation models
- gaussian processes
Fuente:
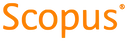
Tipo de documento:
Conference Object
Estado:
Acceso restringido
Áreas de conocimiento:
- Optimización matemática
- Optimización matemática
- Optimización matemática
Áreas temáticas:
- Análisis