Estimation procedure for reduced rank regression, PLSSVD
Abstract:
This paper presents a procedure for coefficient estimation in a multivariate regression model of reduced rank in the presence of multicollinearity. The procedure permits the pbkp_rediction of the dependent variables taking advantage of both Partial Least Squares (PLS) and Singular Value Decomposition (SVD) methods, which is denoted by PLSSVD. Global variability indices and pbkp_rediction error sums are used to compare the results obtained by classical regression with reduced rank (OLSSVD) and the PLSSVD procedure when applied to examples with different grades of multicollinearity (severe, moderate and low). In addition, simulations to compare the methods were performed with different sample sizes under four scenarios. The new PLSSVD method is shown to be more effective when the multicollinearity is severe and especially for small sample sizes.
Año de publicación:
2016
Keywords:
- partial least squares
- Reduced rank
- Multivariate Regression
- Multicollinearity
- SINGULAR VALUE DECOMPOSITION
- PLSSVD
Fuente:
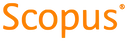
Tipo de documento:
Article
Estado:
Acceso abierto
Áreas de conocimiento:
- Optimización matemática
Áreas temáticas de Dewey:
- Probabilidades y matemática aplicada