An apriori-based learning scheme towards intelligent mining of association rules for geological big data
Abstract:
The past decade has witnessed the rapid advancements of geological data analysis techniques, which facilitates the development of modern agricultural systems. However, there remains some technical challenges that should be addressed to fully exploit the potential of those geological big data, while gathering massive amounts of data in this application field. Generally, a good representation of correlation in the geological big data is critical to making full use of multi-source geological data, while discovering the relationship in data and mining mineral pbkp_rediction information. Then, in this article, a scheme is proposed towards intelligent mining of association rules for geological big data. Firstly, we achieve word embedding via word2vec technique in geological data. Secondly, through the use of self-organizing map (SOM) and K-means algorithm, the word embedding data is clustered to serve the purpose of improving the performance of analysis and mining. On the basis of it, the unsupervised Apriori learning algorithm is developed to analyze and mine these association rules in data. Finally, some experiments are conducted to verify that our scheme can effectively mine the potential relationships and rules in the mineral deposit data.
Año de publicación:
2020
Keywords:
- Apriori
- Association Rules
- Self-organizing map (SOM)
- K-Means
Fuente:
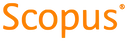