An assessment of ten-fold and Monte Carlo cross validations for time series forecasting
Abstract:
On a meta-learning process, the key is to build a reliable meta-training data set, which requires the best model for a specific sample. In the other hand, the uncertainty of expected accuracy of a particular model increases when data depend on time. Then, during meta-learning, an accurate validation of the reliability of the involved models is critical. This paper compares the applicability of two of the most used methods for validating forecasting models: ten-fold and Monte Carlo cross validations. Experimental results, using time series of the NN3 tournament, found that Monte Carlo cross validation is more stable than ten-fold cross validation for selecting the best forecasting model. © 2013 IEEE.
Año de publicación:
2013
Keywords:
Fuente:
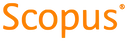
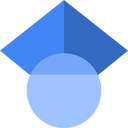
Tipo de documento:
Conference Object
Estado:
Acceso restringido
Áreas de conocimiento:
- Análisis de datos
- Optimización matemática
Áreas temáticas de Dewey:
- Ciencias de la computación