An automatic BI-RADS description of mammographic masses by fusing multiresolution features
Abstract:
Correct mammography evaluation demands great expertise and rigorous interpretation of some radiographic features. The Breast Imaging Reporting and Data System (BI-RADS) is accepted as a standardized method to communicate mammographic findings. This report assigns a detailed description to breast masses in terms of their shape, margin, and density, a process highly dependent on the observer experience. This paper proposes a new automatic BI-RADS characterization of breast masses contained in a Regions of Interest (RoI). For doing so, the proposed approach learns relevant radiological characteristics from several multiscale decomposition of the visual information, the Zernike polynomials and Curvelet bases, which are optimally fused by a Multiple Kernel Learning (MKL) strategy. The method first assesses the presence of masses in the RoI by training a conventional support vector machine (SVM) classifier. Once a RoI is identified as mass-positive, it feeds a bank of SVM binary classifiers that selects the type of shape from the BI-RADS terms. The mass margin and density are then set by combining the BI-RADS labels of the five most similar shapes in the database. Evaluation was conducted using a set of 980 RoIs extracted from the Digital Database for Screening Mammography (DDSM) and 216 RoIs extracted from the INBreast database. Both database descriptions were updated to meet the fifth version of the BI-RADS lexicon. Masses were recognized with a sensitivity of 96.2% and a specificity of 93.1%, while shape, margin and density descriptions showed averaged sensitivity rates between 87.4% and 96.7% and specificity between 85.6% and 96.7%. This results outperformed the obtained when non-fused multiresolution features were used. These results suggest the proposed strategy may be useful in clinical scenarios and may contribute to the training of new radiologists as a second reader.
Año de publicación:
2017
Keywords:
- Zernike moments
- Curvelet transform
- multiple kernel learning
- Mammographic mass description
- BI-RADS mammographic lexicon
Fuente:
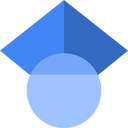
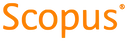
Tipo de documento:
Article
Estado:
Acceso restringido
Áreas de conocimiento:
- Simulación por computadora
Áreas temáticas:
- Enfermedades
- Fisiología humana
- Funcionamiento de bibliotecas y archivos