An automatic recognition of multi-class skin lesions via deep learning convolutional neural networks
Abstract:
An automatic recognition of skin lesions from dermoscopy images is a big challenge task due to the low contrast, a huge inter/intra class variation, and high visual similarity among the different skin lesions. ISIC 2018 classification challenge for dermoscopy images involves seven classes for skin lesions called Melanocytic Nevus (NV), Dermatofibroma (DF), Melanoma (MEL), Actinic Keratosis or Pigmented Bowen’s diseases (AKIEC), Pigmented Benign Keratosis (BKL), Basal Cell Carcinoma (BCC), and Vascular (VASC) lesions. To automatically classify multi-class skin lesions from the dermoscopy images, state-of-the-art deep learning classification models of DenseNet-201, Inception-V3, InceptionResNet-V2, and ResNet-50 are adapted and utilized. The online evaluation results of the validation dataset for skin lesions classification showed multi-class balanced accuracies of 84.60%, 84.70%, 85.80% and 90.10% with DenseNet-201, Inception-V3, InceptionResNet-V2, and ResNet-50, respectively. An automatic recognition for all seven classes of skin lesions could be used to practically assist the radiologists.
Año de publicación:
2018
Keywords:
Fuente:
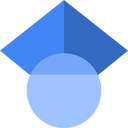
Tipo de documento:
Other
Estado:
Acceso abierto
Áreas de conocimiento:
- Aprendizaje automático
- Ciencias de la computación
Áreas temáticas:
- Métodos informáticos especiales