An efficient recommender system method based on the numerical relevances and the non-numerical structures of the ratings
Abstract:
In this paper, we propose a collaborative filtering method designed to improve the current memory-based prediction times without worsening and even improving the existing accuracy results. The accuracy improvement is achieved by combining the numerical relevance of the ratings with non-numerical information based on the votes structure. The improvement of the prediction time is achieved by setting four actions: 1) simplification of the similarity measure design, in order to minimize the necessary calculations; 2) construction and maintenance of a model that simplifies the predictions processing; 3) optimization of the computation, using a set-based model and a bit-based processing implementation; and 4) switching between the bit processing and the numerical processing, depending on the density of the users' ratings. Experimental results show the improvements both in the prediction time and the accuracy. Experiments have used a significant amount of state-of-the-art baselines and collaborative filtering public data sets.
Año de publicación:
2018
Keywords:
- Similarity Measures
- performance
- recommender systems
- COLLABORATIVE FILTERING
- pbkp_rediction time
- model-based methods
Fuente:
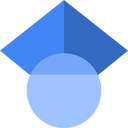
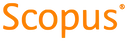
Tipo de documento:
Article
Estado:
Acceso abierto
Áreas de conocimiento:
- Aprendizaje automático
- Ciencias de la computación
- Ciencias de la computación
Áreas temáticas de Dewey:
- Funcionamiento de bibliotecas y archivos

Objetivos de Desarrollo Sostenible:
- ODS 9: Industria, innovación e infraestructura
- ODS 17: Alianzas para lograr los objetivos
- ODS 8: Trabajo decente y crecimiento económico
