An ensemble machine learning based approach for constructing probabilistic PV generation forecasting
Abstract:
Photovoltaic (PV) generation forecasting plays an important role in accommodating more distributed PV sites into power systems. However, due to the stochastic nature of PV generation, conventional point forecast methods can hardly quantify the uncertainties of PV generation. Being capable of quantifying uncertainties, probabilistic forecasting tools, like pbkp_rediction intervals (PIs), are receiving increasing attention. This paper proposes a new framework to construct PIs and make point forecasts. In the proposed framework, an efficient and robust algorithm is employed to perform quantile regression. Based on the quantile regression results, PIs for multiple confidence levels are constructed utilizing different quantiles. Simulation results on a PV generation system reveal that the proposed framework is more reliable and accurate, compared with state-of-the-art methods, as measured by multiple performance indices.
Año de publicación:
2018
Keywords:
- Stochastic gradient boosting machine
- probabilistic forecasting
- Photovoltaic
- Quantile regression
- pbkp_rediction interval
- UNCERTAINTY
Fuente:
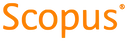
Tipo de documento:
Conference Object
Estado:
Acceso restringido
Áreas de conocimiento:
- Aprendizaje automático
- Energía renovable
- Simulación por computadora
Áreas temáticas:
- Ciencias de la computación