An improved multi-class spectral clustering based on normalized cuts
Abstract:
In this work, we present an improved multi-class spectral clustering (MCSC) that represents an alternative to the standard k-way normalized clustering, avoiding the use of an iterative algorithm for tuning the orthogonal matrix rotation. The performance of proposed method is compared with the conventional MCSC and k-means in terms of different clustering quality indicators. Results are accomplished on commonly used toy data sets with hardly separable classes, as well as on an image segmentation database. In addition, as a clustering indicator, a novel unsupervised measure is introduced to quantify the performance of the proposed method. The proposed method spends lower processing time than conventional spectral clustering approaches. © 2012 Springer-Verlag.
Año de publicación:
2012
Keywords:
Fuente:
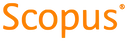
Tipo de documento:
Conference Object
Estado:
Acceso abierto
Áreas de conocimiento:
- Aprendizaje automático
- Algoritmo
- Ciencias de la computación
Áreas temáticas:
- Métodos informáticos especiales