An improvement of reinforcement learning approach for permutation of flow-shop scheduling problems
Abstract:
The tasks scheduling problem on linear production systems, Flow Shop Scheduling Problem, has been of a great importance in Operations Research. This paper addresses the problem of minimizing the completion time of all jobs, known in literature as makespan or C max . This study presents an adaptation of a Reinforcement Learning approach known as Q-Learning which is combined with a local search to improve the solutions. The approach employs a NEH heuristic to give a potential starting solution in the search space and finally we applied the improvement through the different local search methods. An evaluation of different parameters combinations of the algorithm is also given. To validate the quality of the solutions, we test our approach on several standard Taillard’s benchmark problems and the algorithm effectiveness is compared against eight other advanced techniques. The results show that the proposed algorithm reaches high-quality solutions in short computational times.
Año de publicación:
2019
Keywords:
- scheduling
- Optimization
- Q-Learning
- Flow-shop
- makespan
Fuente:
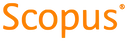
Tipo de documento:
Article
Estado:
Acceso restringido
Áreas de conocimiento:
- Inteligencia artificial
- Algoritmo
- Algoritmo
Áreas temáticas:
- Programación informática, programas, datos, seguridad