An unsupervised approach to geographical knowledge discovery using street level and street network images
Abstract:
Recent researches have shown the increasing use of machine learning methods in geography and urban analytics, primarily to extract features and patterns from spatial and temporal data using a supervised approach. Researches integrating geographical processes in machine learning models and the use of unsupervised approaches on geographical data for knowledge discovery had been sparse. This research contributes to the ladder, where we show how latent variables learned from unsupervised learning methods on urban images can be used for geographic knowledge discovery. In particular, we propose a simple approach called Convolutional-PCA (ConvPCA) which are applied on both street level and street network images to find a set of uncorrelated and ordered visual latent components. The approach allows for meaningful explanations using a combination of geographical and generative visualisations to explore the latent space, and to show how the learned representation can be used to pbkp_redict urban characteristics such as street quality and street network attributes. The research also finds that the visual components from the ConvPCA model achieves similar accuracy when compared to less interpretable dimension reduction techniques.
Año de publicación:
2019
Keywords:
- Knowledge Discovery
- Computer Vision
- Machine learning
- convolutional neural networks
- Urban analytics
- unsupervised learning
Fuente:
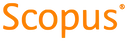
Tipo de documento:
Conference Object
Estado:
Acceso restringido
Áreas de conocimiento:
- Visión por computadora
Áreas temáticas:
- Ciencias de la computación
- Métodos informáticos especiales
- Funcionamiento de bibliotecas y archivos