Analysis of Meta-Features in the Context of Adaptive Hybrid Recommendation Systems
Abstract:
The difficulty in finding the most suitable recommendation algorithm for all requests is a common challenge in the recommendation system context, regardless of the domain. Although the combination of multiple techniques in a hybrid approach is often a good solution, the majority of implementations follow a static hybridization scheme, which does not consider the available data, the user specificities at a given time, or the changing environment. Thus, another challenge is the definition of adaptive hybrid recommenders that are capable to adjust the combination of the recommendation algorithms based on the properties of the dynamic inputs. For that purpose, it is necessary to define meta-features that contain information to effectively differentiate recommendation algorithm behaviors over time, and capture how properties of the user/items are related to their performance. This work studies these meta-features in order to analyze their characteristics, relevance, behavior, redundancy and their abilities to represent the system dynamics over time. The paper presents several experiments that can be used as a meta-feature's evaluation guide, and briefly propose its utilization in a hybrid fuzzy system for recommendation.
Año de publicación:
2022
Keywords:
- Metafeatures in Recommendation systems
- Hybrid Recommendation systems
- Feature engineering
Fuente:
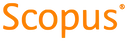
Tipo de documento:
Conference Object
Estado:
Acceso restringido
Áreas de conocimiento:
- Aprendizaje automático
- Ciencias de la computación
Áreas temáticas:
- Funcionamiento de bibliotecas y archivos