Analysis of different affective state multimodal recognition approaches with missing data-oriented to virtual learning environments
Abstract:
In this work, the affective state of users in virtual learning environments is assessed/recognized in terms of continuous arousal and valence dimensions, making use of multimodal information (audio, text and video), whenever any of these modalities are available. In general, virtual learning environments where these three modalities are all the time, are not common; at some moments only the video modality is available, while in others only text or/and video and/or audio. Different approaches using feature-level fusion and decision-level fusion are proposed for multimodal recognition with missing data. Recognizing according to available modalities is studied following the ideas of dropout from neural networks and of variable input length from recurrent neural networks. This proposal is innovative because it represents emotions in the continuous space, which is not common in virtual education; and makes use of the available modalities in a virtual environment in a given moment, which is very common in virtual learning environments because the people are not speaking or writing all the time.
Año de publicación:
2021
Keywords:
- Multimodal recognition
- Affective state
- Arousal and valence dimensions
- Emotional Recognition
Fuente:
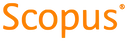
Tipo de documento:
Article
Estado:
Acceso abierto
Áreas de conocimiento:
- Tecnología educativa
- Inteligencia artificial
Áreas temáticas de Dewey:
- Ciencias de la computación
- Psicología aplicada
- Escuelas y sus actividades; educación especial

Objetivos de Desarrollo Sostenible:
- ODS 4: Educación de calidad
- ODS 3: Salud y bienestar
- ODS 9: Industria, innovación e infraestructura
