Analysis of machine learning techniques for the intelligent diagnosis of Ni-MH battery cells
Abstract:
This paper presents a comparison of different machine learning techniques for classification of the unbalance and damage Niquel-Metal Hydride (Ni-MH) battery cells used in hybrid electric vehicles (HEV) and electric vehicles (EV). The implemented linear and non-linear classification algorithms used in this study are: logistic regression (LR), k-nearest neighbors (k-NN), kernel space vector machine (KSVM), Gaussian naive Bayes (GNB) and a neural network (NN); the classifiers in this work used the principal component analysis (PCA) in dual variables to reduce the high dimensional data set. To evaluate the performance of the classifiers, experimental results and a detailed analysis of the confusion matrix are used where the effectiveness of the algorithms are demonstrated.
Año de publicación:
2019
Keywords:
- Ni-MH
- Classifier
- Hybrid electric vehicle
- Machine learning
Fuente:
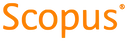
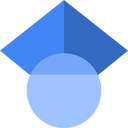
Tipo de documento:
Conference Object
Estado:
Acceso restringido
Áreas de conocimiento:
- Energía
- Aprendizaje automático
- Simulación por computadora
Áreas temáticas:
- Ciencias de la computación
- Física aplicada
- Métodos informáticos especiales