Evaluation of downscaled estimates of monthly temperature and precipitation for a Southern Ecuador case study
Abstract:
The downscaling of global climate models (GCMs) aims at incorporating finer scale information to their horizontal resolution in order to represent regional and local processes better. There are two main approaches to downscaling: statistical (based on data relationships between synoptic atmospheric variables and observations of local variables) and dynamical (based on the modelling of regional atmospheric processes and land-surface interactions). In this study, some pbkp_redictive capabilities regarding the generation of station-scale mean monthly temperature and rainfall of both a statistical artificial neural network (ANN-based) and a dynamical weather research and forecasting (WRF-based) downscaling approach are assessed. We have devised two versions of the statistical downscaling approach. One of them includes regional orographic variables as pbkp_redictors to allow for spatial extrapolation; the other is purely local. Historical observational data, from the period 1990 to 1999, of two major watersheds in the Ecuadorian Southern Andes, the Jubones and Paute river basins, were used. Since, to a certain extent, the value added by downscaling techniques can be attributed to terrain information, it is worth noting that some characteristics of the selected catchments (as notorious altitude differences and the presence of qualitatively different precipitation regimes) provide a scientifically interesting location for exploring how finer scale effects are captured. For this reason, we concentrate on the ability of downscaling techniques to reproduce seasonality. A decade of evaluation proved that both approaches were able to qualitatively describe precipitation and temperature seasonal variations for different regimes at representative weather stations. Furthermore, the seasonality of precipitation represented by both downscaling approaches surpassed the seasonality representation of reanalysis data. However, shortcomings on the estimates were found. Specifically, dynamical downscaled precipitation estimates were prone to overestimation. Despite the fact that the considered downscaling approaches are different in nature, their ability to represent the high spatio-temporal variability in this region highlights the importance of evaluating their strengths and limitations.
Año de publicación:
2016
Keywords:
- Dynamical downscaling
- WRF
- ECUADOR
- RCM
- Statistical downscaling
- Artificial neural network (ANNs)
Fuente:
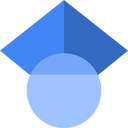
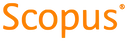
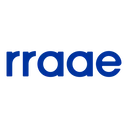
Tipo de documento:
Article
Estado:
Acceso restringido
Áreas de conocimiento:
- Clima
- Clima
Áreas temáticas:
- Geología, hidrología, meteorología