Evolutionary robust optimization in production planning – interactions between number of objectives, sample size and choice of robustness measure
Abstract:
We aim to find robust solutions in optimization settings where there is uncertainty associated with the operating/environmental conditions, and the fitness of a solution is hence best described by a distribution of outcomes. In such settings, the nature of the fitness distribution (reflecting the performance of a particular solution across a set of operating scenarios) is of potential interest in deciding solution quality, and previous work has suggested the inclusion of robustness as an additional optimization objective. However, there has been limited investigation of different robustness criteria, and the impact this choice may have on the sample size needed to obtain reliable fitness estimates. Here, we investigate different single and multi-objective formulations for robust optimization, in the context of a real-world problem addressed via simulation-based optimization. For the (limited evaluation) setting considered, our results highlight the value of an explicit robustness criterion in steering an optimizer towards solutions that are not only robust (as may be expected), but also associated with a profit that is, on average, higher than that identified by standard single-objective approaches. We also observe significant interactions between the choice of robustness measure and the sample size employed during fitness evaluation, an effect that is more pronounced for our multi-objective models.
Año de publicación:
2017
Keywords:
- Robust optimization
- Evolutionary multi-objective optimization
- Simulation-based optimization
- Production planning
- Uncertainty modelling
Fuente:
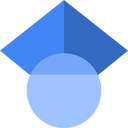
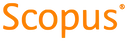
Tipo de documento:
Article
Estado:
Acceso restringido
Áreas de conocimiento:
- Optimización matemática
- Optimización matemática
- Optimización matemática
Áreas temáticas:
- Dirección general