Explainable Model of Cbkp_redit Risk Assessment Based on Convolutional Neural Networks
Abstract:
Cbkp_redit Risk Assessment estimates the probability of loss due to a borrower’s failure to repay a loan or cbkp_redit. Therefore, one of the principal challenges of financial institutions is to lower the losses generated by leading financial resources to possible default clients. Current models for Cbkp_redit Risk Assessment used by the industry are based on Logistic regression (LR), thanks to their operational efficiency and interpretability. However, Deep Learning (DL) Algorithms have become more attractive than conventional Machine Learning due to their best general accuracy. However, Models for Cbkp_redit Risk Assessment based on DL have a problem because their complexity makes them difficult for humans to interpret. Additionally, international regulations for financial institutions require that models be interpretable. In this work, we propose the use of a model based on Convolutional Neural Networks (CNN) and SHapley Additive exPlanations (SHAP) to generate a more accurate and explainable model than LR models. In order to demonstrate its efficacy, we use four datasets commonly used to benchmark classification algorithms for cbkp_redit scoring. The results show that the method proposed is more accurate than LR for large datasets (more than 5900 samples), with an improvement in accuracy up to 12.3%.
Año de publicación:
2022
Keywords:
- Explainability
- DeepInsight
- Cbkp_redit risk assessment
- cnn
Fuente:
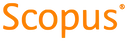
Tipo de documento:
Conference Object
Estado:
Acceso restringido
Áreas de conocimiento:
- Red neuronal artificial
- Ciencias de la computación
Áreas temáticas:
- Programación informática, programas, datos, seguridad
- Economía
- Física aplicada