Exploiting generative adversarial networks as an oversampling method for fault diagnosis of an industrial robotic manipulator
Abstract:
Data-driven machine learning techniques play an important role in fault diagnosis, safety, and maintenance of the industrial robotic manipulator. However, these methods require data that, more often that not, are hard to obtain, especially data collected from fault condition states and, without enough and appropriated (balanced) data, no acceptable performance should be expected. Generative adversarial networks (GAN) are receiving a significant interest, especially in the image analysis field due to their outstanding generative capabilities. This paper investigates whether or not GAN can be used as an oversampling tool to compensate for an unbalanced data set in an industrial manipulator fault diagnosis task. A comprehensive empirical analysis is performed taking into account six different scenarios for mitigating the unbalanced data, including classical under and oversampling (SMOTE) methods. In all of these, a wavelet packet transform is used for feature generation while a random forest is used for fault classification. Aspects such as loss functions, learning curves, random input distributions, data shuffling, and initial conditions were also considered. A non-parametric statistical test of hypotheses reveals that all GAN based fault-diagnosis outperforms both under and oversampling classical methods while, within GAN based methods, an average accuracy difference as high as 1.68% can be achieved.
Año de publicación:
2020
Keywords:
- random forest
- Fault diagnosis
- Unbalance data
- Feature Extraction
- Generative Adversarial Network
Fuente:
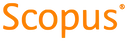
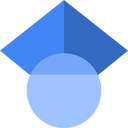
Tipo de documento:
Article
Estado:
Acceso abierto
Áreas de conocimiento:
- Software
- Aprendizaje automático
Áreas temáticas:
- Física aplicada
- Métodos informáticos especiales