Exploratory Study of Physic Informed Deep Learning Applied to a Step-Pool for Different Flow Magnitudes
Abstract:
Physical laws governing a certain phenomenon can be included in a deep-learning model within a new paradigm: the so-called physical informed deep learning (PIDL). Physical laws in hydraulics consist of partial differential equations (PDEs) resulting from balance laws. The potential use of PIDL in a step-pool reach having a complex flow and geometric characteristics is tested in this article. The studied morphology belongs to a hydraulic observatory in a mountain river in Ecuador where flow and geometric data are available. The water level profile of PIDL was compared to a stationary one-dimensional HEC-RAS model and water levels measured at three staff gauges in the reach. Saint–Venant equations, geometry data, and boundary conditions were used to implement a PIDL-based model. The chosen PIDL architecture is based on the one with the lowest value for the loss function. The resulting water level profile of the PIDL model does not have instabilities, and according to dimensionless RMSE is slightly less efficient in its pbkp_redictions than the HEC RAS model. Moreover, the difference between HEC-RAS and PIDL water profile decreases as flow increases.
Año de publicación:
2022
Keywords:
- Physics Informed Deep-Learning
- Mountain River
- Field data
- Step-pool
Fuente:
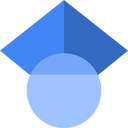
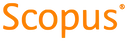
Tipo de documento:
Conference Object
Estado:
Acceso restringido
Áreas de conocimiento:
- Simulación por computadora
- Aprendizaje automático
- Dinámica de fluidos
Áreas temáticas:
- Métodos informáticos especiales
- Ingeniería y operaciones afines
- Ciencias de la computación