Anova and cluster distance based contributions for feature empirical analysis to fault diagnosis in rotating machinery
Abstract:
The number of extracted features for fault diagnosis in rotating machinery can grow considerably due to the large amount of available data collected from different monitored signals. Usually, feature selection or reduction are conducted through several techniques proposing a unique set of representative features for all available classes; nevertheless, in feature selection, it has been recognized that a set of individually good features do not necessarily lead to good classification. This paper proposes a general framework to analyse the feature selection oriented to identify the features that can produce clusters of data with a proper structure. In the first stage, the framework uses Analysis of Variance (ANOVA) combined with Tukey's test for ranking the significant features individually. In the second stage, a further analysis based on inter-cluster and intra-cluster distances, is accomplished to rank subsets of significant features previously identified. In this sense, our contribution aims at discovering the subset of features that are discriminating better the clusters of data associated to several faulty conditions of the mechanical devices, to build more robust fault multi-classifiers. Fault severity classification in rolling bearings is studied to test the proposed framework, with data collected from an experimental test bed under real conditions of speed and load on the rotating device.
Año de publicación:
2017
Keywords:
- Fault diagnosis
- bearings
- cluster validity assessment
- Anova
- Feature engineering
Fuente:
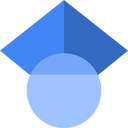
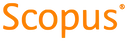
Tipo de documento:
Conference Object
Estado:
Acceso restringido
Áreas de conocimiento:
- Aprendizaje automático
Áreas temáticas:
- Física aplicada
- Métodos informáticos especiales