Factorization techniques for longitudinal linked data
Abstract:
Longitudinal linked data are RDF descriptions of observations from related sampling frames or sensors at multiple points in time, e.g., patient medical records or climate sensor data. Observations are expressed as measurements whose values can be repeated several times in a sampling frame, resulting in a considerable increase in data volume. We devise a factorized compact representation of longitudinal linked data to reduce repetition of same measurements, and propose algorithms to generate collections of factorized longitudinal linked data that can be managed by existing RDF triple stores. We empirically study the effectiveness of the proposed factorized representation on linked observation data. We show that the total data volume can be reduced by more than 30% on average without loss of information, as well as improve compression ratio of state-of-the-art compression techniques.
Año de publicación:
2016
Keywords:
- Longitudinal linked data
- Data factorization
Fuente:
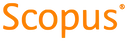
Tipo de documento:
Conference Object
Estado:
Acceso restringido
Áreas de conocimiento:
- Análisis de datos
Áreas temáticas:
- Funcionamiento de bibliotecas y archivos