Factors that Affect i-Vectors Based Language Identification Systems
Abstract:
The performance of a language identification (LID) system that uses i-vectors as features depends on several parameters, such as algorithm parameters and data parameters. In this study, an analysis of performance of a language identification system is considered, for which we focused only on data parameters in the “Back End” of the system, analyzing the influence of the amount of data and the speaker variability in the training phases of the UBM and the total variability Matrix T. Also, the Multiclass logistic regression (MLR) classifiers were analyzed, by balancing the classes of the database to train the classifiers on each language. These tests have been carried out in the Kalaka-3 database; we have used the average detection cost function (Cavg) to evaluate the performance. It is shown experimentally that in the training phase of the UBM, speaker variability is more important than a large amount of data. In the training phase of the total variability matrix T a better performance was obtained when a larger number of audios were used. And finally, balancing classes on each language to train the MLR classifiers allowed us to get a better performance only in certain languages. Using all of these proposed variations, we got a Cavg improvement of 37% in a standard language identification system.
Año de publicación:
2020
Keywords:
- Language Identification
- i-Vector
- DATA
Fuente:
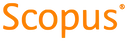
Tipo de documento:
Conference Object
Estado:
Acceso restringido
Áreas de conocimiento:
- Aprendizaje automático
- Ciencias de la computación
Áreas temáticas:
- Funcionamiento de bibliotecas y archivos